DOI: 10.25136/2409-8698.2023.8.43875
EDN: VMTZYT
Received:
22-08-2023
Published:
05-09-2023
Abstract:
This article is devoted to the study and analysis of modern technologies, namely artificial intelligence as an auxiliary tool in the work of a translator. The purpose of the study is to find out whether chatbots based on GPT–3.5 technology can be used in translation activities. The article compares the capabilities of chatbots and online text corpora, as well as checks their analytical abilities and ability to rhyme. Dialog assistants are given a task to find information or analyze it based on a query given to them. At the same time, it is checked whether chatbots developed by different companies provide the same information. The scientific novelty of the work lies in the attempt to find a universal tool that can automate the routine work of the translator. A special contribution of the authors of the study is an attempt for the first time to compare and analyze the rapidly developing functionality of chatbots in the context of translation activities and to identify key problems that do not allow the effective use of this technology in translation. As a result, it was determined that the modern GPT language model has many limitations and disadvantages that stop chatbots from becoming a reliable source of information and an efficient translation tool. Problems were identified, the solution of which would make it possible to use Chat GPT and other chatbots in translation activities.
Keywords:
chatbot, artificial intelligence, Chat GPT, Computer-Aided Interpreting systems, neural networks, text corpora, pre-translation analysis, Sage, Perplexity, Chatsonic
This article is automatically translated.
Introduction. To date, achievements in the field of artificial intelligence have long been used in translation activities [1, p. 51]. The ability to work with Computer-Assisted Translation programs is a necessary translator skill and a requirement of many employers, as this technology greatly facilitates the translation process. Over time, Computer-Aided Interpreting systems have been developed – programs for interpreters that take some of the load off them and allow them to focus on the meaning of words. These programs are based on Translation Memory, Machine Translation and Speech Recognition technologies. Naturally, at the moment in translation, artificial intelligence is used to perform routine work, which usually takes a lot of time [8, p. 264; 3, p. 112]. However, there are still tasks in the translator's work that we have to face regularly, and the solution of which could be automated with the help of chatbots using ChatGPT technologies. Such tasks include checking the frequency of a certain word or phrase, searching for collocations, comparing synonyms and paronyms, searching for rhymes, pre-translation analysis. We assume that chatbots can combine a number of Internet resources, which would allow them to become a universal tool in the arsenal of a modern translator. The purpose of this study is: 1) conducting a review of research on chatbots using ChatGPT technologies, their application in translation and some related fields; 2) conducting an empirical study to test the ability of chatbots to provide the translator with reliable linguistic information and solve other translation tasks; 3) find out how different the quality of information provided by chatbots using OpenAI technologies is, depending on who developed them; 4) identification and discussion of key issues related to the effectiveness of the use of chatbots in translation activities. Literature review. To begin with, let's look at what a "chatbot" is. There are many definitions of this concept, but they all note only those characteristics that are important for a particular field of application. So O. S. Bikulova and M. I. Ivkina, who are engaged in teaching Russian, give the following definition: "A chatbot is a computer program created to simulate a real dialogue between a virtual interlocutor (artificial intelligence) with a user" [2, p. 92]. A.V. Kopytova, considering the features of communication between artificial intelligence and a person, defines a chatbot as "a program whose purpose is to interpret the input text or speech and output a corresponding useful response" [4, p. 124]. The chatbot B. Luo, Raymond Y. K. Lau, C. Li and Y. V. Si are understood as "smart dialog agents that interact with users in natural languages" [11, p. 2]. A. N. Korobova and N. D. Chizhik, considering the chatbot as an assistant to the applicant when Upon receipt, it is defined as "a special program that facilitates the user's life, simplifying the search for necessary data and helping in solving issues" [5, p. 71]. Within the framework of this study, we are not so much interested in the ability of a chatbot based on artificial intelligence to simulate a dialogue, as in the ability to quickly search for information, analyze it, collect it and present it to the translator in a convenient form. It is these characteristics that are noted by A.V. Kopytova and A. N. Korobova. Therefore, based on the above definitions and in relation to the topic of translation using computer systems, it is proposed to understand a chatbot as "a program that searches for data and helps in solving questions, first interpreting the input message, and then outputting a useful answer, interacting with the user in natural language in the form of a dialogue" [7, c. 23]. To date, the most popular chatbot with artificial intelligence is ChatGPT, developed by OpenAI. GPT stands for Generative Pre-trained Transformer, which means "generative pre-trained transformer". ChatGPT is a language model that is trained on a huge array of data. He is able to write code in an interactive mode, create texts, translate and give answers to a variety of questions. Most chatbots use ChatGPT version 3.5 technology, which was trained on data until September 2021. That is, any changes that have occurred in the languages for 2022-2023 will not be taken into account by the neural network when issuing responses. M. V. Proshina examines the use of neural networks in natural language processing and identifies question-answer systems and intelligent analysis. The results of the study relate to the topic of this study, since chatbots combine a question-and-answer system and intelligent analysis [6, p. 28]. The article notes that neural networks can generate answers that are both true and absolutely incorrect. This remark is very important, since one of the goals of our research is to find out whether chatbots can be a reliable source of information for translators.
By performing intelligent text analysis, artificial intelligence "mechanically extracts information from all kinds of text data, such as books, scientific journals, clinical records, news articles, posts and comments, and so on" [5, p. 70]. Thus, chatbots should have a huge amount of textual information, which in theory allows them to more fully reflect natural languages than specialized text corpora. It is worth mentioning the tests conducted by NewsGuard regarding ChatGPT 3.5 [6]. As a result of verifying the accuracy of the information provided by ChatGPT, it was found out that the answers may depend on the language in which the request was entered (prompt), since for a certain language the chatbot is trained on the corpus of this particular language. A linguistic corpus is a large array of texts collected in accordance with certain principles, marked up according to a certain standard and provided with a specialized text and linguistic data management system. To date, the enclosures are computer-based, that is, presented on a specific machine medium, which greatly simplifies the work of researchers. R. Firaiana and D. Sulisvoro came to the conclusion that, although ChatGPT has some limitations, it can be a good alternative in training, since the lecturers acting as respondents in the study confirmed that using a chatbot increased their productivity and efficiency in finding new information [9, p. 44]. M. Javaid, A. Khaleem, R. Pratap Singh, Sh. Khan and I. Khaleem Khan argue that due to its extensive capabilities, such as, for example, providing entire lists of terms on a specific topic and their meanings, ChatGPT can be a very powerful tool both in improving the level of knowledge of students and in the work of teachers [10, p. 9]. Similar studies on the use of Chat-GPT for the most part in the field of education prove the logic of trying to find a use for chatbots in translation activities. Materials and methods. The empirical study was conducted on the basis of the Department of Linguistics and Translation of the Institute of Humanitarian Education of the Moscow State Technical University named after G.I. Nosov in 2023. Its purpose was to test the ability of three Sage, Perplexity and Chatsonic chatbots based on GPT-3.5 technology to effectively perform the functions of translation assistants, searching for information and solving some other translation tasks, as well as to determine what problems and issues GPT and chatbot developers need to solve in order to make use of dialog assistants effective. As part of the study, chatbots needed to give the required response to a request formed in the form of a text message. Prompta were formed in order to solve the following translation tasks: - determination of the frequency of use of the token; - search for collocations; - comparison of paronyms; - search for synonyms; - selection of rhymes; - pre-translation analysis of the text. Research results 1. Determining the frequency of use of the word. This section presents the results of an experiment conducted with three Sage chatbots on the platform Poe.com , developed by Quora; Perplexity AI, developed by a small team of Perplexity; and Chatsonic from Writesonic. Although all three use GPT-3.5 technologies, each has its own characteristics. We will make identical or similar promptas in order to find out at the end which type of chatbot best copes with translation tasks. And the first task that chatbots had to cope with was to determine the frequency of the word. There are text corpora for this, but our task was to find out if it could be done using a chatbot. The word "pollution" was chosen for the search, which occurs 19195 times in the COCA case. Chatbots needed to find out how often this word is used in the American version of English. Figures 1-3 show that not a single chatbot was able to give a useful answer to the question. While Perplexity AI and Chatsonic refused to give an exact figure, even having a specific source where such information is contained, Sage came up with it altogether. The first chatbot did not even provide an approximate frequency of use of the word. We will discuss the topic of false information in more detail in the relevant section. 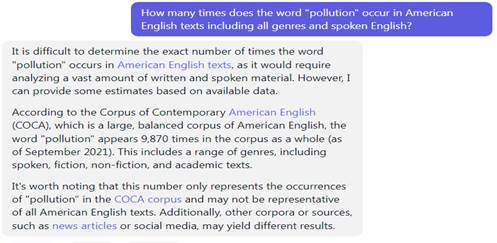 Fig. 1. Checking the frequency of use of the word in the Sage chatbot. 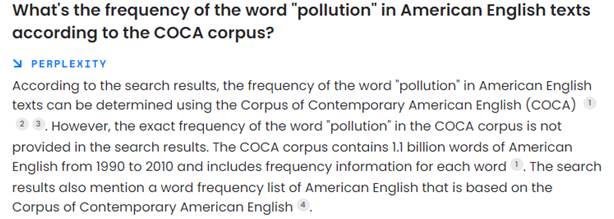
Fig. 2. Checking the frequency of word usage in the Perplexity AI chatbot. 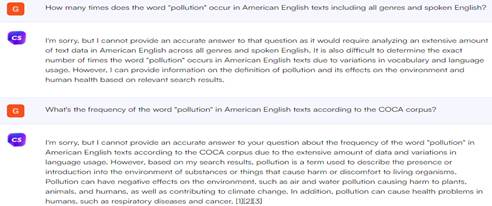 Fig. 3. Checking the frequency of use of the word in the chatbot Chatsonic. 2. Search for collocations. In addition to the frequency of use of a particular lexical unit, translators need to check the compatibility of words from time to time in order to avoid mistakes. So, chatbots needed to provide a list of 10 words that are most often used with the word "pollution". The list should have been compiled in such an order, where in the first place is the word that makes up the collocation with "pollution" more often than all the others. In Figure 4, we see that Sage has again turned to the COCA corpus, but his answers do not correspond to reality. Both in COCA and in the British English BNC corpus, the adjective "environmental" is not in the first place. The results in Fig. 5-6 were taken by chatbots from the site the Adjective Finder. However, no exact figures or even the number of texts based on which this list was compiled can be found on the site. The information obtained with the help of chatbots can still be useful only if the sole purpose of the translator is to find out what collocations exist with a word, or to find collocations in order to better understand the shades of the meaning of the lexeme. Roughly speaking, chatbots do not fulfill the requirement of this request. 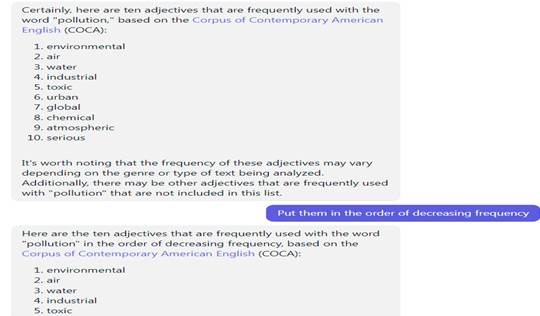 Fig. 4. Search for collocations in the Sage chatbot. 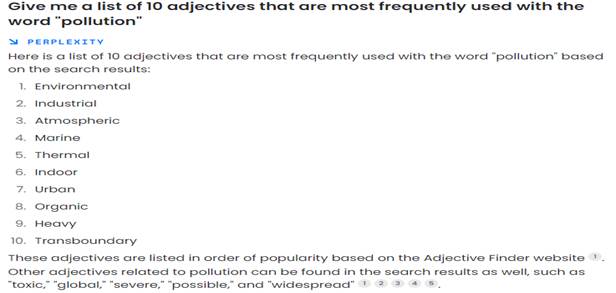 Fig. 5. Search for collocations in the Perplexity AI chatbot. 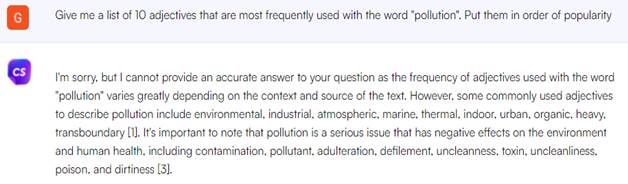 Fig. 6. Search for collocations in the chatbot Chatsonic. 3. Comparison of paronyms. The translator, of course, must know the paronyms not only in his native language, but also in the language with which he works. However, sometimes you have to compare two words in order to better understand what their differences are and in what contexts they are used. This time, chatbots needed to explain the difference between the paronyms "sensitive" and "sensible", as well as provide access to the full texts, as many corpora do. In Figure 7. Sage really explained the difference between words and gave examples of how to use them in texts. However, in order to get access to the full texts, it was necessary to request links to them, which we did in the second clarifying question. The fact that you don't have to write prompta completely anew is a feature of OpenAI chatbots that remember your past messages. Thus, even if a mistake has been made, you can always fix it in the next request. Perplexity AI usually doesn't need to be clarified that we need links to the source texts, but we did it anyway. Figure 8-9 shows that opposite each sentence there is a link to the source from where it was taken. But none of the links were useful for one of three reasons: 1) the link is non-working; 2) there was no such sentence with the given word in the text; 3) the sentence was taken from an online dictionary, and not from the real text. As for Chatsonic, it provided only 3 links, which for the same reasons turned out to be useless (Fig. 10). 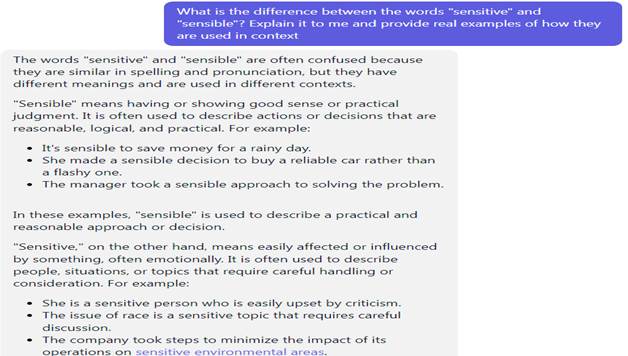 Fig. 7. Comparison of two paronyms from the Sage chatbot 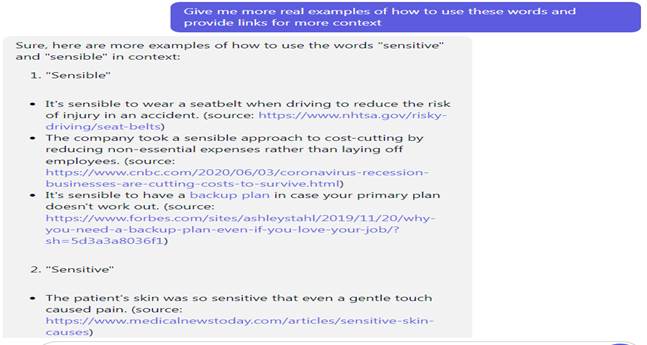 Fig. 8. Requesting links from the Sage chatbot. 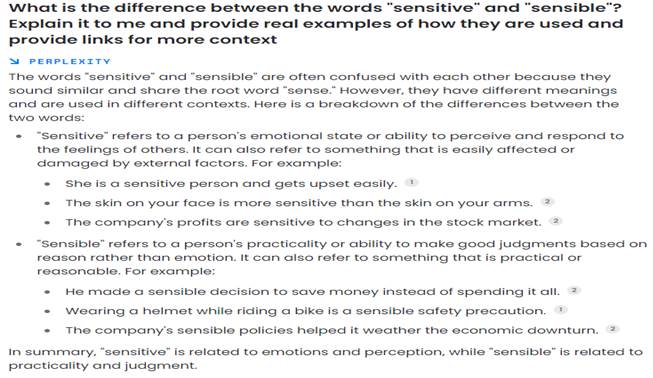 Fig. 9. Comparison of two paronyms from the chatbot Perplexity AI. 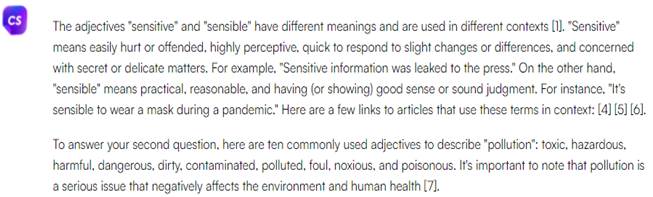 Fig. 10. Comparison of two paronyms from the chatbot Chatsonic. We suggested that it might be better to indicate where the chatbot needs to take examples from, be it the media or literature. However, this attempt was not crowned with success. Chatbots give examples, indicate the names and authors of works, but we found that there are actually no such sentences in the texts (Fig. 11). Every single example was generated by a neural network, and not obtained from real sources.
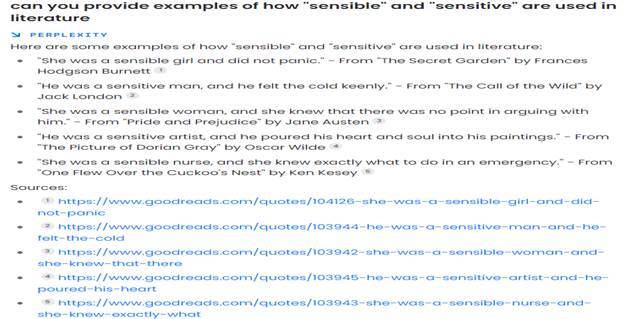 Fig. 11. Examples from the literature from the chatbot Perplexity AI. 4. Search for synonyms. A translator should always replenish his vocabulary in order to be able to find a suitable equivalent in a variety of situations. Due to ignorance of synonyms of the same concept or insufficient training, the translator may make stylistic mistakes. We decided to check how well chatbots are able to select synonyms. In Fig. 12-13, we see that they proposed many options. Perplexity AI even gave examples of their use, and Chatsonic left links to dictionary entries. 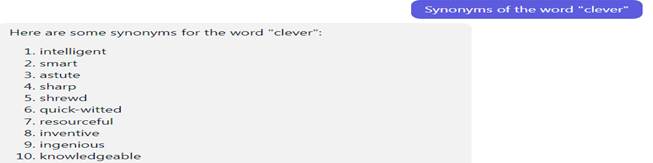 Fig. 12. Synonyms from the Sage chatbot. 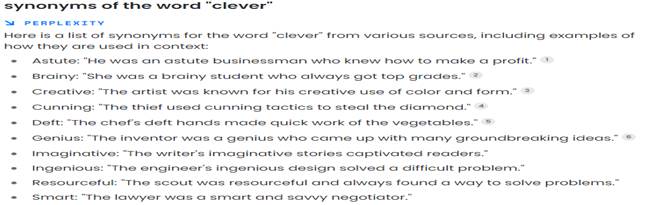 Fig. 13. Synonyms from the Perplexity AI chatbot. 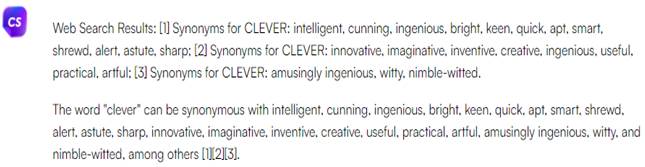 Fig. 14. Synonyms from the chatbot Chatsonic. After that, we decided to check whether chatbots can find synonyms for the same word that relate to formal vocabulary (Fig. 15-17). In addition to "intelligent", we received words such as "astute", "ingenious", "adroit" and "resourceful", which really belong to the formal vocabulary, if guided by the COCA corpus. 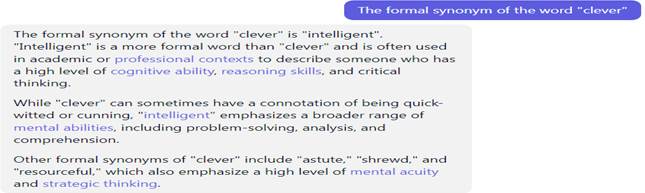 Fig. 15. Formal synonyms from the Sage chatbot. 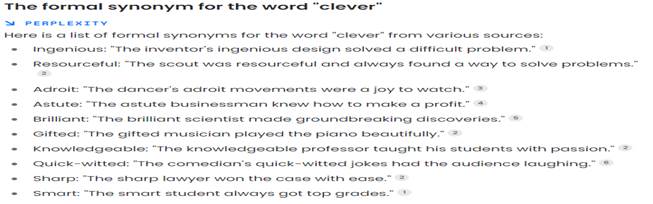 Fig. 16. Formal synonyms from the chatbot Perplexity AI. 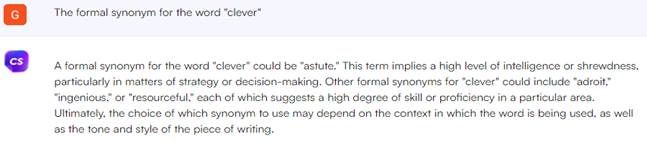 Fig. 17. Formal synonyms from the chatbot Chatsonic. 5. Selection of rhymes. The next task was the practical application of chatbots in the translation of poetry. We are not talking about machine translation using neural networks, as this is considered unacceptable for quite fair reasons. We assumed that chatbots can select the most appropriate rhymes to the already translated string. Translating poetry, translators have long used special sites that give out lists of rhymes to a given word. The fact that chatbots are able to take into account the context could be their advantage, since in theory they could select from all possible rhymes those that best fit the meaning. For the experiment, we took a poem, on the example of which the Russian linguist, translator and professor D. I. Ermolovich in the 6th issue of the video cycle "Linguistic subtleties" on the website www.youtube demonstrated how a poetic translation is created. Chatbots needed to find a word for the third line that would rhyme with the first one, which D. I. Ermolovich translated as follows: "The soul is tired – does not want changes." Sage picked up the words "harmful" and "vain", but they do not rhyme with the first line, since in them the stress falls on the first syllable, and not on the last (Fig. 18). Perplexity AI failed to find a rhyme for the word "change" based on the original. But the chatbot can give a list of rhyming words that are not related in meaning (Fig. 19). The short adjective "bezmen", which was suggested by Chatsonic and which actually means "huge, immeasurable", does not fit at all, although it rhymes with the first line (Fig. 20). 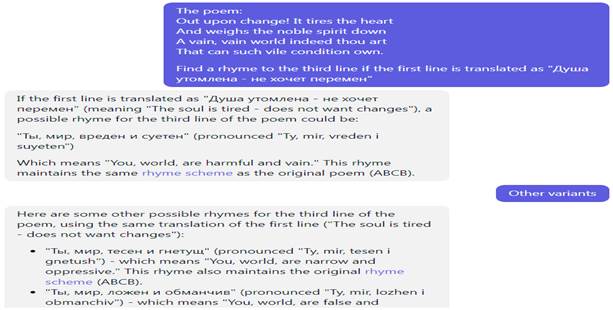 Fig. 18. Selection of rhymes from the Sage chatbot. 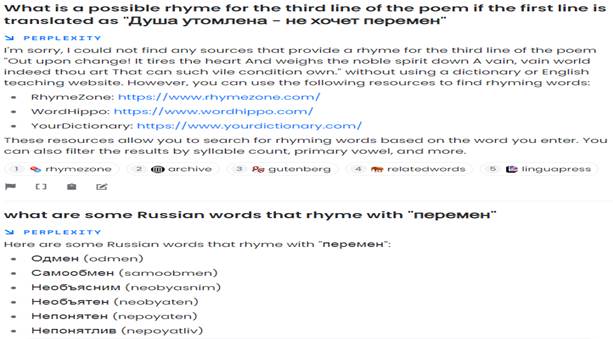 Fig. 19. Selection of rhymes from the chatbot Perplexity AI. 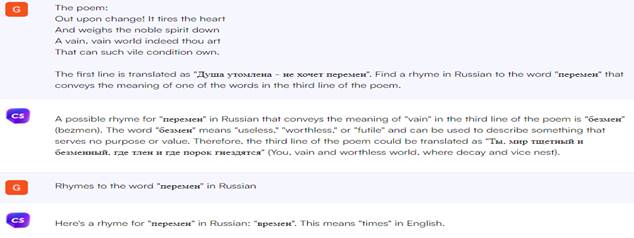 Fig. 20. Selection of rhymes from the chatbot Chatsonic.
6. Pre-translation analysis of the text. Pre-translation text analysis is an integral part of the translator's work. However, this is routine work that can be automated. Chatbots needed to answer the questions asked based on an excerpt from the text, which would help the translator better understand what text he was working with. We took the first excerpt from Mark Twain's novel "Tom Sawyer", without specifying the title or any unnecessary information. The results were different, and the best answer was given by the Sage chatbot (Fig. 21). Having correctly identified the author and the title of the work, he easily found the date of publication of the text and its genre. The answers to the other questions were also correct. Perplexity AI identified the author, the title, but he could not find the publication date (Fig. 22). Also, the answer to question 4 about the target audience does not give us any useful information ("The target audience of the text is the readers of the book "The Adventures of Tom Sawyer"). Chatsonic could not determine the date of publication of the text and accurately determine the genre of the text (Fig. 22). 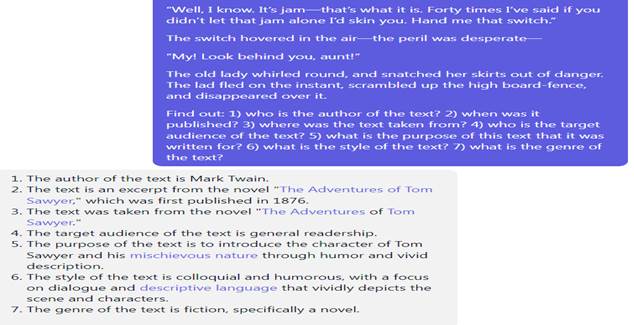 Fig. 21. Pre-translation analysis of "Tom Sawyer" from the Sage chatbot. 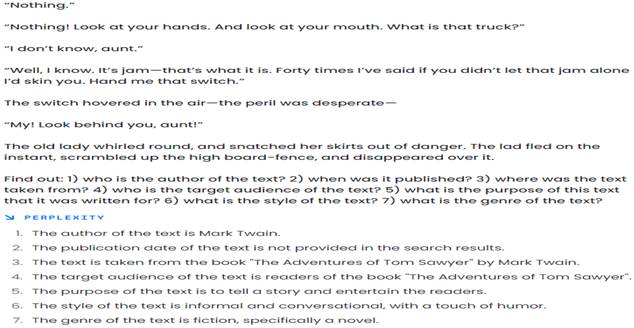 Fig. 22. Pre-translation analysis of "Tom Sawyer" from the chatbot Perplexity AI. 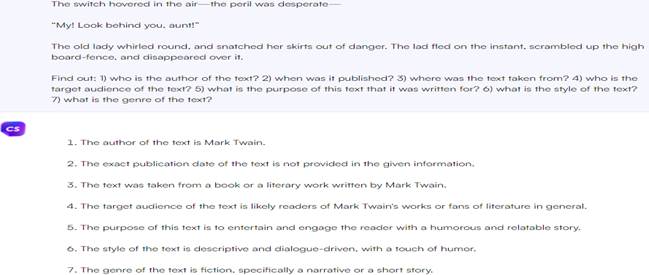 Fig. 23. Pre-translation analysis of "Tom Sawyer" from the chatbot Chatsonic. The following text for analysis was taken from the CNN news site. This time, no chatbot was able to set the date of publication or the author (Fig. 24-27). According to OpenAI, GPT-3.5 has only data up to September 2021, which may be the reason why neural networks could not determine the publication date. Therefore, news related to 2019 was found about forest fires in Australia and repeated questions were asked. As we can see in Figure 25, the chatbot cannot determine the author of the text, the date or the source. The other two chatbots were also unable to answer these questions. Perplexity AI claims that the text was taken from the South China Morning Post resource (Fig.26). It is unlikely that the same text was actually published on this site, since less than a day has passed since its publication in CNN, and the link provided by Perplexity AI turned out to be non-working. Chatsonic could not analyze the text at all and requested the source from where the information was taken (Fig.27). 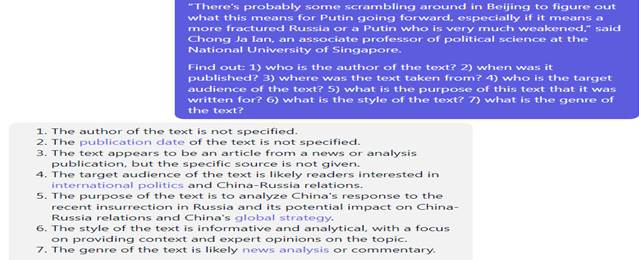 Fig. 24. Pre-translation analysis of the journalistic text from the Sage chatbot. 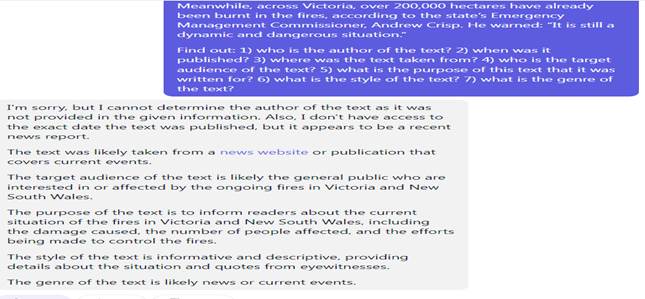 Fig. 25. Pre-translation analysis of the 2019 text from the Sage chatbot. 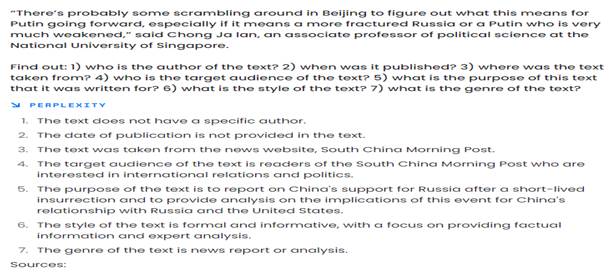 Fig. 26. Pre-translation analysis of the journalistic text from the chatbot Perplexity AI. 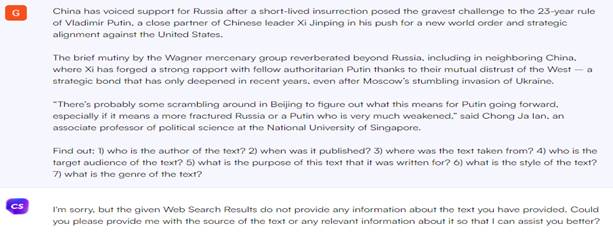 Fig. 27. Pre-translation analysis of the journalistic text from the chatbot Chatsonic. Discussion of the results. 1. The quality of the information provided. The results of the study confirmed M. V. Proshina's statement that neural networks can generate answers that are not only true, but also absolutely incorrect. All three chatbots repeatedly turned to unverified sites during the search or to non-existent sources, even when the necessary information was freely available, as in an experiment with comparing two paronyms. They may incorrectly determine the meaning of the word. The only time when the chatbots' responses fully satisfied the request was the search for synonyms. In this case, they turn to dictionaries and can even correctly determine the case of words, despite the fact that even the most proven dictionaries do not always indicate this characteristic. Not always accurate answers were given to requests for text analysis. Thus, chatbots can really quickly search for information, no matter what the request is, however, in order to get reliable data, you still have to turn to traditional search methods. 2. The ability to analyze information and other capabilities.
It turned out that despite the fact that GPT-3.5 was trained on a huge array of linguistic data, chatbots for some reason cannot count how many times a word was used in texts. One of them turned to the COCA English language corpus, but gave out false information, which suggests that the chatbot, unable to use the corpora, generated the figure itself. A similar situation occurred with the search for words in context, when chatbots referred to special sites to search for quotes, from where they allegedly found sentences. On these sites, we could not find the same examples given by chatbots, which means they really cannot use the functions of other sites. It is not entirely clear why generate non-existent information when there are many open sources where it is not necessary to use the functions of the site to find words in the text. Most chatbots can correctly identify the genre of a text and even its purpose, but not the publication date or source. However, any translator can just as well answer these questions themselves by reading the text fluently. Chatbots made attempts to find a rhyme for the word, but they did not take into account the accents in the words. Nevertheless, a useful feature of chatbots remains the ability to supplement their past requests by clarifying them. 3. Which chatbot is better? Let's start with the fact that the most inefficient chatbot turned out to be Chatsonic. He did not break the information into paragraphs, which made it difficult to perceive the text. In addition, of the three chatbots, Chatsonic gave the least examples and sources, while the search for an answer took an average of 1-2 minutes. Although the developers claim that he can use up-to-date Google information in search, his answers were not more useful than those of the other two chatbots. In the process of working with Chatsonic, I had to face errors more than once and re-enter the request. In addition to all this, Chatsonic was unable to analyze the text, even when all the necessary information for answering questions was freely available. Sage and Perplexity AI were equally convenient to use: the information is broken down by meaning, each example is numbered, quick answers. Nevertheless, we prefer the Sage chatbot, so its text analysis turned out to be the most complete. The disadvantage of Perplexity is its advantage, namely that it always leaves links to where the information was taken from. When a question concerns some non-obvious information or information that the chatbot does not know, it generates its own, but continues to leave non-working links. 4. Chatbot to replace dictionaries and text corpora: convenience in work. The very idea of conducting research or solving translation problems without the help of any complex programs sounds attractive. However, there are two points here. On the one hand, it is much easier to use one tool, and make all queries in simple language instead of learning the functionality of several tools in order to effectively search for information. On the other hand, having learned to use, for example, corpora, the translator needs to perform fewer actions and at the same time get high-quality information. Using a chatbot, the user is forced to take into account everything that can affect the answer and express it with words. As a result, the preparation of the prompta may take too much time for the translator. In addition, chatbots cannot provide access to the full text, while in the corpus it is almost always possible to get a broader context. It is worth noting that they were well able to explain the difference between the words "sensitive" and "sensible", also look for synonyms, so in theory, given that they leave links to dictionary entries, they can be used instead of dictionaries. Conclusions. Dialog assistants have their pros and serious cons. The quality of the information provided, as well as the sources to which artificial intelligence refers, depends on the company that developed the chatbot. They are still unable to solve most translation tasks, and their ability to increase efficiency and productivity in the search for information, which R. Firaiana and D. Sulisvoro claim, remains in doubt. Any information obtained with the help of chatbots needs to be checked for authenticity, which takes a lot of time. The GPT-3.5 language model, which is currently used by most chatbots, does not allow them to become one of the tools in the translator's arsenal. It is still more convenient to access different resources during the translation process than to make a long request taking into account possible misunderstandings on the part of artificial intelligence. Nevertheless, chatbots still have potential, and they can become useful in their work if the following problems are solved: a) falsification of data; b) inability to use the search functions of other sites; c) inability to calculate the frequency of use of words and expressions in texts; d) indication of links to non-existent sources; e) lack of consideration of accents in words when selecting rhymes; f) inability to access the full texts.
References
1. Artamonova, M. V. (2022). CAT tools in training future translators. Current issues of modern science, technology and education, 13(2), 50–53.
2. Bikkulova, O. S., & Ivkina, M. I. (2021). Chatbots in teaching Russian as a foreign language. Mir Russkogo slova, 1, 91–96. doi:10.24412/1811-1629-2021-1-91-96
3. Zilberman, N. N. (2007). The use of chatbot technologies in the formation of speaking skills in the teaching of a foreign language. Humanitarian Informatics, 3, 110–116.
4. Kopytova, O. V. (2023). Linguopragmatical aspects of communication situation “human–chatbot”. Human: image and essence. Humanitarian aspects, 2(54), 123–139. doi:10.31249/chel/2023.02.07
5. Korobova, A. N., & Chizhyk, N. D. (2000). Use of chatbots as additional assistants for prospective students MRK. In V. V. Shatalova, M. A. Belchik, & E. A. Lazytskas (Eds.). Scientific conference of college students: materials of the 58th scientific conference of postgraduate, graduate and undergraduate students of GGUIR (pp. 70–73). Minsk: BSUIR.
6. Proshina, M. V. (2022). Modern methods of natural language processing: neural networks. Economics of construction, 5, 27–42.
7. Hobson, L., Hannes, H., & Cole, H. (2020). Natural language processing in action. St. Petersburg: Peter.
8. Aleksandrova, E. V., Trofimova, N. A., Rubtsova, S. Yu., & [et al.]. (2023). Audiovisual content analysis in the translation process. Journal for Educators, Teachers and Trainers, 14(3), 262–268. doi:10.47750/jett.2023.14.03.032
9. Firaina, R., & Sulisworo, D. (2023). Exploring the Usage of ChatGPT in Higher Education: Frequency and Impact on Productivity. Buletin Edukasi Indonesia, 2(1), 39–46. doi:10.56741/bei.v2i01.310
10. Javaid, M., Haleem, A., Singh, R. P., Khan, S., & Khan, I. H. (2023). Unlocking the opportunities through ChatGPT Tool towards ameliorating the education system. BenchCouncil Transactions on Benchmarks, Standards and Evaluations, 3(2), 1–12. doi:10.1016/j.tbench.2023.100115
11. Luo B., Lau, Y. K. R., Li, C., Si & Y-W. (2022). A critical review of state-of-the-art chatbot designs and applications. Wiley Interdisciplinary Reviews: Data Mining and Knowledge Discovery, 12(1), 1–26. doi:10.1002/widm.1434
Peer Review
Peer reviewers' evaluations remain confidential and are not disclosed to the public. Only external reviews, authorized for publication by the article's author(s), are made public. Typically, these final reviews are conducted after the manuscript's revision. Adhering to our double-blind review policy, the reviewer's identity is kept confidential.
The list of publisher reviewers can be found here.
The article is devoted to one of the urgent problems of our time – chatbots, which actively occupy effective positions in various spheres of human life. The author of the work is focused on the consideration of these programs in translation mode, which supports the relevance of the study, determines its novelty. As noted at the beginning of the work, "to date, achievements in the field of artificial intelligence have long been used in translation activities. The ability to work with Computer-Assisted Translation programs is a necessary translator skill and a requirement of many employers, as this technology greatly facilitates the translation process. Over time, Computer-Aided Interpreting systems have been developed – programs for interpreters that take some of the load off them and allow them to focus on the meaning of words. These programs are based on Translation Memory, Machine Translation and Speech Recognition technologies." The purpose of the study has been clarified and specified: 1) conducting a review of research on chatbots using ChatGPT technologies, their application in translation and some related fields; 2) conducting an empirical study to test the ability of chatbots to provide reliable linguistic information to the translator and solve other translation tasks; 3) to find out how the quality of information provided by chatbots differs, using OpenAI technologies, depending on who developed them; 4) identification and discussion of key issues related to the effectiveness of using chatbots in translation activities. The work has a completed form, it is competently compiled, the available text volume is enough to reveal the topic. The practical nature of the research is available, it is indicated that "an empirical study was conducted on the basis of the Department of Linguistics and Translation of the Institute of Humanitarian Education of the Moscow State Technical University named after G.I. Nosov in 2023. Its purpose was to test the ability of three Sage, Perplexity and Chatsonic chatbots based on GPT-3.5 technology to effectively perform the functions of translation assistants, searching for information and solving some other translation tasks, as well as to determine what problems and issues GPT and chatbot developers need to solve in order to make use of dialog assistants effective." The fragmentation of the text into so-called semantic blocks allows the potential reader to move after the author, gradually master the material. The style of work correlates with the scientific type itself, the terminological block is used correctly. It is appropriate for the author to include pictograms in the work, they justify the analysis, illustrate the progress of the study. For example, "in addition to the frequency of use of a particular lexical unit, translators need to check the compatibility of words from time to time in order to avoid mistakes. So, the chatbots needed to provide a list of 10 words that are most often used with the word "pollution". The list should have been compiled in such an order, where in the first place is the word that collocates with "pollution" more often than all the others. In Figure 4, we see that Sage has again turned to the COCA corpus, but his answers do not correspond to reality. In both COCA and the British English BNC corpus, the adjective "environmental" is not in the first place. The results in Fig. 5-6 were taken by chatbots from the site the Objective Finder", or "The translator, of course, must know the paronyms not only in his native language, but also in the language he works with. However, sometimes you have to compare two words to better understand what their differences are and in what contexts they are used. This time, chatbots needed to explain the difference between the paronyms "sensitive" and "sensible", as well as provide access to the full texts, as many corpora do. In Figure 7. Sage really explained the difference between words and gave examples of how to use them in texts. However, in order to get access to the full texts, it was necessary to request links to them, which we did in the second clarifying question. The fact that you don't have to write prompta completely over again is a feature of OpenAI chatbots that remember your past messages. Thus, even if a mistake has been made, you can always fix it in the next request. Perplexity AI usually doesn't need to be clarified that we need links to the source texts, but we did it anyway. Figure 8-9 shows that opposite each sentence there is a link to the source from where it was taken," etc. In my opinion, the main goal of the study has been achieved, the tasks set have been solved. The conclusions / results of the text correspond to the main block: "Dialog assistants have their pros and serious cons. The quality of the information provided, as well as the sources accessed by artificial intelligence, depends on the company that developed the chatbot. They still cannot solve most translation tasks, and their ability to increase efficiency and productivity in the search for information, which R. Firaiana and D. Sulisvoro claim, remains in doubt. Any information obtained through chatbots needs to be verified for authenticity, which takes a lot of time. The GPT-3.5 language model, which is currently used by most chatbots, does not allow them to become one of the tools in the translator's arsenal. It is still more convenient to access different resources during the translation process than to make a long request, taking into account possible misunderstandings on the part of artificial intelligence. Nevertheless, chatbots still have potential, and they can become useful in their work if the following problems are solved: a) falsification of data; b) inability to use the search functions of other sites; c) inability to calculate the frequency of use of words and expressions in texts; d) specifying links to non-existent sources e) the lack of consideration of accents in words when selecting rhymes; f) the inability to access the full texts." The work has a completely completed look, it will be interesting and useful for both professionals and just beginning researchers (translators).The requirements of the publication are taken into account, the text does not need serious editing and correction. I recommend the article "Chatbot as a tool in the work of a translator" for publication in the magazine "Litera".
|